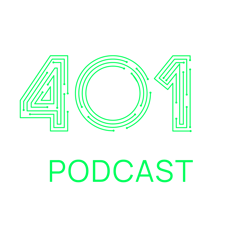
Joseph Carson:
Hello everyone. Welcome back to another episode of the 401 Access Denied Podcast. I'm the host, Joe Carson, Chief Security Scientist and advisory CISO here at Delinea. It's a pleasure to be here with you today. We have a very important topic, one that's been a bit of a buzzword for the last couple of years and it's really exciting, but also has some risks and challenges and we're going to cover those today in the episode. And I'm actually welcome with an amazing guest. So welcome to the episode today, Dr. Andrea Isoni. If you can give us a little bit of background of who you are, what you do, and some of the fun facts about yourself.
Andrea Isoni:
Hi everyone. Thank you for having me. I'm Andrea Isoni and I'm Chief AI Officer of AI Technologies, a company that developed data related product for essentially other companies. And yeah, I'm been involved within machine learning and data science for a decade now, I'll say. And fun fact about myself, I wanted to be a Formula One engineer and just change career.
Joseph Carson:
Well, it's definitely, you maybe still have that possibility at some point.
Andrea Isoni:
Well, yeah.
Joseph Carson:
There's always the future. Welcome to the show and the episode today, we really want to dive into a couple of things. One is that AI has been a big topic mostly in the last few years. But when I look at it, it's been a big topic around for the last probably 50, 60 years because it's a topic it's been looked at, but I think where it's become much more available to the wider public in the last few years and that's where the trend has really coming from. But when we get into AI itself, there's lots of challenges around ethics and around acceptable use and so forth. First of all, let's explain to the audience what really is AI because I think AI in general, from a very broad topic, is misunderstood and I think it's really important to really give the fundamentals about what AI. Is and then we can talk a little bit more about details of ethics and the risks.
Andrea Isoni:
AI is just a software, the main difference with any other software you've react with is this software has a bunch of data or certain sort, certain thing, has been trained, that's the word. I don't know. I'm trying to figure out how to simplify the word trained, but there's nothing coming to my mind. But in any case, it means that these software takes some data, try to understand some statistical feature or pattern into this data. That's what we call training. Okay. And then based on that, based on the pattern you learn when you interact with it, will figure out if any pattern is already learned is applicable to you or to the question you ask you, ask it.
Joseph Carson:
Okay, so simplicity, it's much more contextual aware. It understands that. I always call it's probably more about predictive. It's from what you're asking, it's starting to learn about what's the next thing that it should be actually presenting to you. And the more questions you ask, the more that tries to understand the context of what you're asking and learning from that. And ultimately for me, I think from the audience and I always call it, it's literally it's fast math. It's-
Andrea Isoni:
Fast statistics.
Joseph Carson:
Exactly. Because what it's able to do is based on lots of, and your point is, one of the challenges is that when we have those large language models and ultimately, you end up having the machine learning type of thing where it starts learning the context of those. I did a lot of work in the past. One of the challenges I had was around many years ago I worked in projects which was looking at natural language understanding muscle and natural language processing because that's the convergence between human speak and machine speak. And that really allows you to really start understanding about what's the context of the question being asked in that natural language, and then computing that into computer understanding. Many years ago I did work was around the mid, early nineties, which was a language translator, which was some of the early use cases was to translate languages in a much more effective way. What's some of the practical uses that you've seen? We've seen the ChatGPT and we've seen other types, but what's the more practical examples that you've seen AI being used in, in use cases?
Andrea Isoni:
In which sector industry?
Joseph Carson:
An. Let's say even... Probably in the medical probably has been the most that's been used in recent years due to things like COVID where it's been used to accelerate, let's say, medicine discovery.
Andrea Isoni:
Yeah. Oh, definitely. You just touched a topic now I can talk about, well I can talk in any way. It was a big probe. Yeah, definitely, there are new patent or drug et cetera. There have been, if anything accelerate or entirely discovered, essentially a large trained AI tool. DeepMind, I forgot it just leap in my mind the name, but there is a big tool from DeepMind that actually discover some patents or some new drug or proteins. Yes, some proteins. Just using some big neural network model.
Joseph Carson:
Mm-hmm. And for the audiences from neural networks is really where you got many computers working together to solve a problem and they all divide up how they're going to do that in a large scale so they can do it much faster. And that's one of the things that from using neural networks and AI algorithms, because ultimately, it comes down to it's literally an algorithm. It's an algorithm that has data, that has some type of context that we're in training and every time it processes, it learns new ways of looking at that data, statistical, from a not math perspective. Now, when we talk about ethics, because that's one of the big challenges.
I've sat in numerous expert panels and boards in recent years and it goes way back, a lot of my working in this was around 2015 to 2020 in some of the early discussions around things like the EU AI act, which we'll talk in a little bit now. One of the things was there's lots of ethical challenges. One of the things is that a lot of the AI models that we have today are biased by default because the data sets that have been used is based on data that has been historical and if you look at a lot of the data that we've got, it's historical biased. So can you explain some of the examples of those types of bias or ethical challenges that we have with AI algorithms today?
Andrea Isoni:
Yeah, just to start with some simple example, you probably, I will not go to the monkey one, but I will go to a more professional one probably. I'm sure you know what the monkey is if one I can say even that, but just to go into, it's the same problem anyway. So essentially, let's say you train a model on historical data on salaries to predict salary of a person based on age, gender, race or whatever it is.
You build a model out of that and then you use the model in a prediction phase essentially. Me, Andrea Isoni, 37, white, clearly, and then it will predict a certain range of salary. Now because of the way it's been trained, or the data it's been trained, if a woman goes there, it doesn't matter if exactly the same qualification, I mean essentially my same CV, but it's not male, you see a female, you will predict a lower range of salary. The reason for that is because historically Google will pay less no matter if you match the CV. Yeah.
That's an example of a bias on data is not because... By the way, artificial intelligence is probably, we should say, more unethical, there's no ethic that not embedded in the system. Yeah.
Joseph Carson:
Correct.
Andrea Isoni:
None of that. It just-
Joseph Carson:
It's not the algorithm itself, it's the data that's actually biased. It's not the algorithm. The algorithm will not know the difference.
Andrea Isoni:
Not sentient. It's not sentient, it's not judging you, it's not doing any of that. Okay. It is just the way it discovered frankly is our fault because the data comes from us.
Joseph Carson:
We created the data and during that historical time is that there's been times of the past where things are not equal, things are not earned at that stage. And it's really important that what we try to do is not use the data from the past but use the potential data that will be the future. And I think that's the way that, we have to reverse this around is that using the data from the past but actually starting to think about, well what should it be in the future, not use the data to predict. Because I did things in the past, for example, using algorithms to predict when a disc space will run out or when memory will be exceeded on a server and you use the historical data in the past in order to do that. But what we had to be able to do is use the present understanding to your point is the ethical understanding of today, and not try to use the ethical understanding of the data of the past because they are very different. To your point, to things like gender bias.
We even have hemisphere bias as well because most of the data that we're using today has basically from technology advanced countries, which much of that has been basically in the Northern Hemisphere. So we're actually missing a huge amount of knowledge and data input from the Southern Hemisphere. And this is going to also create bias built in as well. And also gets into your point is you end up with pay discrepancies, gender discrepancies, religion, and it's getting to the point where it's really getting difficult into where do we let the AI model have freedom of decision before we intercept with some type of human oversight or human intervention.
So even from the data models itself, I've seen also challenges about using it in law. We saw cases in, I think it was New York State, where a lawyer basically created a deposition based on an AI. What's your thoughts around, one is the ethics from a data perspective, but also what uses, should we also be very careful such as using it in the legal system as well? Should we be very careful and what's your thoughts about what areas should we be very cautious or maybe move it a bit slower pace in some cases?
Andrea Isoni:
Okay, there it's two questions. So on the ethical side, et cetera, you're right, you shouldn't extract it because in the example I was saying, essentially that was extracted from the previous data. So you didn't invent it, it was just extracted as, again, it's a statistical, you say male, more pay grade, male more pay in the future. That's what it was doing, no more than that. And what you're saying is actually create data, are using a little bit more technical term, which I'm sure you're familiar with is synthetic data. It's kind of a big topic today, which means essentially as you say, you or us as human, you define an ethics, you fix that as in male and female paid same. You fix that as a principle, which means an assumption in the code, whatever, and then you derive a statistical distribution, okay, whatever. And then you generate data based on that.
And by the way, then you check later because in the generation you can even use AI to generate data and you check that all the assumption you wanted are in the data. This is super important, especially in certain industry. The reason why we know, I've been I exposed a lot on synthetic data is because some, well, in cybersecurity you need synthetic data. The reason is essentially, you want to predict or try to avoid or prevent tax, accurate tax they're not yet in the data. They're new.
Joseph Carson:
Yes. What you know is what's expected from historical data, the normal and operations, what you're not trying to do is look-
Andrea Isoni:
But the accurate will try new things.
Joseph Carson:
Exactly. And you're looking for the new, for things that hasn't been done in the past. This is new. It's never been done before in your environment.
Andrea Isoni:
That's why there use a lot of synthetic data.
Joseph Carson:
And also, I also think as well when we talk about ethics as well, I like a lot of the Estonian approach because I'm based in Estonia and one of the things is that in the language in Estonian, they don't have any gender, in the language. So when you think about that is that, when we look at algorithms, should we try to remove the things that create bias? So removing those attributes, should salary be done based on skill, not gender? So gender should not be an allocated part of it.
So getting to the point where you're able to extract anonymous types of predictions rather than base, where those types of attributes when you think about religion and age and gender, all of those things that can create biases that we should start excluding them from some of the predictions so that it doesn't become a part of an ethical challenge because that data has now been extrapolated.
Andrea Isoni:
Yeah, that's definitely one way to do it. Obviously, it's case dependent. It depends also if necessary to have gender inside that, for example, for medical, et cetera probably shouldn't do that.
Joseph Carson:
Yeah, in medical there's differences of course, absolutely. If we're talking about surgeries then-
Andrea Isoni:
It depends on the case. Yes, obviously, if you not try to predict a disease or something is important to understand if you're female, male or whatever, just a simple example of that. But if you come back to the second part of the question, I think you asked about in the legal system what can be done, not done. Well, we have actually approval counsel done in Saudi Arabia for the Ministry of Justice to predict essentially judgment on car accident or things like that.
So my opinion is that there are pros and cons in everything. If you structure it correctly, which means you kind of avoid the bias of gender, stuff like that because you structure that way. So this is our responsibility to structure. If you did that, to do that, definitely when you use system like that and the cases in the case, the legal case are standardized. What I mean by that, a car accident, I mean it's kind of a standardized kind of boring case. Someone got hit or crashed, whatever there is the picture, there is the evidence or there isn't. And so he's trying to claim something that is. I mean you see, when you're going a criminal case or something like that, that is wild west, what is true, not true. The variability of the case.
Joseph Carson:
I think you're absolutely right. When you're getting into the point between what's mathematical possible and what's basically, let's say human nature, you can separate those two because AI is purely from a math perspective and it's math reduction, it's about statistical analysis. And if you basically focus on cases that are purely mathematical calculation versus that trying to determine the motive of it within somebody's mind, those are two very separate types of approaches-
Andrea Isoni:
Correct.
Joseph Carson:
... Because the human mind, then you're talking about kinetics and all the different possibilities that we don't know which can't be done with AI at this time.
Andrea Isoni:
Well yeah, I mean there is too much unknown, unknown, non unknown, et cetera. The variability is too large, the AI cannot not make. But if you using a sense in cases which the variability is within control, as I say, car accidents, things like that, which the variability is well-defined and within a range, then I think it's useful. It is useful because first of all you have a standardization of judgment because we are all human. Even the judge is a human being. There was a funny story, if you allow me, it was very quickly.
Joseph Carson:
Sure. Absolutely.
Andrea Isoni:
There is a statistic, I don't remember which country, unfortunately that doesn't matter, on judges' judgment before lunch, after lunch. It lunch, yeah. Is even at work, is afternoon work compared to before lunch and they found out that after lunch was always statistically a bit more gentle, I don't know, in the judgment than before lunch. So this kind of bias, all these kind of bias can be obviously eliminated if you use statistical system, it doesn't have to have lunch system.
Joseph Carson:
Yeah. It's consistent.
Andrea Isoni:
Yes, correct. It's consistent. Although you need to use first of all as we say before, the statistical bias should be eliminated from the top. But if you do that, then you have a very consistent judgment and obviously you need to use for casing, which you know already the variabilities. I mean car accident okay is to fine to fine. We have the evidence? Yes? No? Is there damage proportionate to the amount ask? Yes? No? Go for it, that's it. But yeah, that's the way. So it's not... Even the ethical is too broad. You need to split in sector in which you think AI can help. I think that it's still beyond.
Joseph Carson:
Yeah, I think it really comes down to is because I'll say this, it is maths. I remember having a great discussion a couple of years ago. We were at a hotel and it was after a conference and me and a friend of mine were talking about quantum computing and AI and all of those kind of, the buzzwords at the time. And we literally come down to was literally it was about, getting to the point, it's about fast math as reducing it down to asking the right question and getting it down to as quickly as possible to possible outcomes. So to your point, when you're looking at certain cases that it's all about basically the evidence and that evidence can point to a certain outcome. You can do it much faster if you put it through maths, rather than humans trying to determine all the possibilities. But when you get into emotion, that's the different separation is the emotional aspect of it or the gray area where it's about people's motives or why and assumptions and based on hearsay.
I think that's where really still the humans have a lot to play on. So one thing I got upset last year during a specific event and it was when the organizer came out and the house was introducing the events. And they said the image that you see in the background here was created using AI, and then the music that you're listening to is created using AI. And ultimately, the choreography of the activity that was happening was created using AI. For me it was like well someone created the algorithm, somebody wrote something that ultimately put some type of random pieces of code in there, that results in this.
Are we starting to misunderstand about accountability and responsibility? Because I think when we are saying that AI did it, is that when things go wrong, who is ultimately responsible because then we can point to the AI and say it was their fault, they did it. So is there, at some point in time, where does accountability come in here when it makes a mistake or also when you get into copyright or you get into data rights management issues or you think about royalties, how much of it did it change using the algorithm itself to create this new output?
So what's your thoughts around that aspect of... When it comes, it's really about the arts and sciences and data protection, data rights side of things that I think it's a bit...
Andrea Isoni:
Yeah. Okay, responsibility come at the end or accountability on that, for that matter. So whoever essentially pull the trigger and use the AI and then is responsible to whatever the output is into a real life... he has the responsibility to essentially... Because it's a chicken, we need to understand that this is a kind of a chicken problem. People will think, let's say, I coming to the question or not too much, let's say developer not needed because the GPT will do all the work. The developer not needed. Technically right maybe. But the problem is who check that the software now is correct? So there you need still people that understand code to understand the machine. So a chicken and a problem, yes it can be better but then you are oblivious... or not.
Joseph Carson:
You'll end up with the same bug over and over again because ultimately, the bug was consistent.
Andrea Isoni:
Until someone gets the knowledge. There we go. And then that's exactly the feedback loop. What I mean using your example. So essentially, you are oblivious this thing cuts the bug over and over until someone understand it as the knowledge and then he fix it. My ear is the fake, this now fix the new discovery, now is a data point that you put in the system. Now trained again the bug is not there anymore. Now here's the thing, the person that found the bug is essentially needs to be rebutted for that, in the same way as you say the artist or the IP or the image you get retributed for. That's why I talk in my newsletter of the hacker economy because if you do not attribute people that discovered these bugs, more or less like in the blockchain you have the validator that unlock the chain.
Joseph Carson:
The miners.
Andrea Isoni:
The miners, yes, it's that mentality, but as you know the miners are retributed. You do not retribute the miners, the system will not improve.
Joseph Carson:
Absolutely you're going to end up with the same mistakes over and over again because ultimately, it's humans who created this.
Andrea Isoni:
The IP law comes from that, just to close the loop, the IP and the protection is because essentially it's the way to you to retribute you. That's the IP for. There's no other reason to have an IP on because you need a mechanism to retribute through like hey, I discover that, I deserve to be paid. Something like that. Please go on.
Joseph Carson:
Yeah, no for me, absolutely. It comes down to some type, at the end of the day the algorithm is created by somebody. And that then the algorithm has evolved and the question is that how has it evolved? What's the contribution or the content that has been added to that algorithm to make it more intelligent, make it more automated, make it more improved. And ultimately we humans, we don't create perfection, we create, otherwise the whole software industry is full of bugs and we're going to create bugs in this, and the algorithm itself is not going to fix the bugs. Maybe it will, but ultimately somebody has to go through quality control, somebody has to check it, somebody has to validate it and we have to keep improving it to the point where we get it to as best as we can because ultimately, it's not going to be perfection.
We can just make sure we can do it as best as it can to capture all of the scenarios. And it gets into also the decision when we think about autonomous cars, is that when autonomous cars are going to have an accident is what decision, which car makes the decision in order to reduce the potential victims or casualties as much as possible. And this gets really into, that's another challenge that we have is that when there's a human life at the end of it, when does the algorithm get to make the final decision? Because it can make the decision much faster than we can, which is important because if we are left to make the decision, then ultimately the worst accident could potentially be the outcome, because we're too late to react. So in certain situations handing it over to algorithms to do it faster, but the question is from who makes the decision, which is more valuable than not.
Andrea Isoni:
This becoming... I think it should be still the human, but that means the human still catch up with knowledge, which means you need to have as much as knowledge is possible, no more. If you want to solve someone else problem or a bug in the system, it means you know more than the system itself.
Joseph Carson:
Yep. And that ultimately means that at some point in time the country's laws will actually have to be able to capture these in a legal system as well, into what is the outcomes as what should the final decision be made and therefore that should then be brought back into the algorithms based on the legal frameworks that's out there. So let's move into one of the things I think it's really important is many countries have taken different stances on this. The US is a bit undecided, it's still quite open, anything can go. The UK has created the best practice framework along with CISA. So it's more of a guideline. And EU has really taken a much more governance stance, let's say, by looking at the EO AI Act.
So what's your thoughts around the EU AI Act itself? Is it something that... And the differences as well between different countries as approaches because I always find it's better to be more cautious because fixing things later, we know in security when you try to secure things much later, many bad things have already happened. And you want to do secure by default, you want to do secure by design and I think this is the stance with different approaches. So can you talk about the EUAI Act in general? What's its intentions in order to achieve and what's also the negatives as a result of having something like the EU AI Act place?
Andrea Isoni:
Okay, I'll try to be as brief as possible because this is a very big thing-
Joseph Carson:
It is.
Andrea Isoni:
... Especially related to the other countries, things like that. But very quickly the main principle that EU AI act is about protecting life. It's not about, obviously reflect to business ... relate to business, but the main point is regulate something that affect, impact lives in any way or form, ban something that is again similar, weapon obviously or things like that or which means that biometric, that distinguished by race et cetera in public place or take information about race or gender, whatever it is, ban or things like that, is allowed within system by high tier, high regulator within private compound, the need to emotional reaction with certain jobs and that yes, it's still allowed obviously by high regulator. High regulator is obviously everything related to our care or judgmental system or even education is very high regulated, high risk tier because if the AI got wrongly the wrong score in your education exam, there's a high impact in your future care.
Joseph Carson:
Yes, absolutely.
Andrea Isoni:
Health care is obvious. I'm not getting to that.
Joseph Carson:
It makes the wrong prescription.
Andrea Isoni:
Obviously, its the obvious.
Joseph Carson:
... The wrong doses of drug could be very severe.
Andrea Isoni:
Not going to get into something like that, obviously. And the lower end is something that is more fundamental like chat bot that may or may not use hard language or bad language on your something like that. Yes, it's a problem but it's a live problem on that. It's impacting people, yes, but it's not that much of a problem. So this is different. So ban high tier, high risk, low, middle risk and obviously there is the GBR. That's the structure of EU AI Act.
Joseph Carson:
Which is great. It's just taking a risk-based approach, especially when it comes to my life.
Andrea Isoni:
Yeah, its a risk-based approach, based on impact of life, based on impact of life. Just quickly on that, yesterday there was an approval by the parliament. It's not the end, although the news they say it's the end, it's not the end. I figured there are two steps still in, you can figure out. One is the EU council above the parliament needs to approve that and after that, it needs to enter in the journal. If you any legislation before getting actual law, it needs to be-
Joseph Carson:
Which is everyone has to sit around a table and go-
Andrea Isoni:
Yeah, and then you... Publish in the journal, your journal, I mean the actual whether any country, any law, it needs to be published in the gazette or something like that. There is a name of that. So we are talking about when this gets into actual and fourth, we are talking about May or June. We don't have a specific date around May or June. For that is between six months or banned and banned within six months if you're doing that, you should stop. But for all the rest... depending what it is, it's between 12 and 13, six months and transition period to add. Stop that. Now, the rest of the countries, it's very complicated.
Yes, there light touch in theory. In practice, it's difficult. I explained what I mean by that. First of all, US, UK or other countries rather for example, think that there's no necessity to regulate AI in the sense that is any other any sector, especially UK think that if there is a necessity on regulation, it will be done or pushed by sector. US is even more complicated. It's kind of at the same guidelines but US is as is a United States, which means any state-
Joseph Carson:
Every state is doing their own approach.
Andrea Isoni:
California, for example is doing his own stuff, other are more permissive things like that. So yes, when we are talking US is permissive, you are saying that the federal government is permissive. It's not that any kind of something can do these own laws, et cetera. For example, even in other stuff like environment, things like that, California is super strict regulation on environment, electrical, whatever, other are more relaxed for whatever reason. When we say US, its-
Joseph Carson:
Is, yeah, it depends if its supreme federal level versus state.
Andrea Isoni:
Depends on the state. Federal true state as in single state, not so. UK or other... But anyway, the principle is if any regulation is necessary, is necessary within a sector. So it's regulation by sector. What I mean by that, let's say of finance or cyber sector, if you think in finance there is because finance is already regulated. That's why I say healthcare is already regulated, already a lot of regulation to get in to provide a service. The approach is if they think there is no regulation that already applicable to AI, they need to add within the same sector. Or I travel federal aviation, I'll give you an example aviation because I think it's very easy to understand for everyone. I'm sure everyone look for a flight ticket to somewhere, Joseph from Talin to London where I'm from. You look that Okay, okay, well the price all right. You look for tomorrow and you'll see that price up.
The first thing you say, "They target me because they know that yesterday I look at about data." Its not. They can't. Forget about AI, so before AI they couldn't because there is a regulation that you cannot do arbitrators on a single individual, is banned. If they want to raise a price to Joseph, they right to rise the price to everyone else, just to be clear. That's exactly the reason why UK say, "Okay, you want to use AI to engineer the price up and down? Well there is already a regulation," it's already been, I mean they already using model to statistical model to regulate the price. In this case, do I need a regulation for specific AI model? We already have that. There's no need to have a specific regulation. I hope the point is clear on that. Now, but that's not the end of it. That's where things got interesting. Since you have a vertical approach, which means that any third parties that now provide to different industries, they may need to be regulated.
That's a new technologies, for example. We are develop model for different industries. We are not particularly working. We had client in finance, we don't have any aviation. My fee we would have now with the vertical approach, which seems like touch, we should abide to all the regulation and for each industry, if you want to play in an industry industry. So you see the point, it seems like that touch, eighties again at top level, but when you actually look at it as a third party that before, I mean the responsibility was for the client, not necessarily for all the change. Now you're forcing to every provider to be regulated to any sector which is for each of them. So it seems like but not necessarily.
Joseph Carson:
It's more challenging. Definitely for me-
Andrea Isoni:
Instead of one like EU AI Act, you need all of them.
Joseph Carson:
You need to do all of them. And that's why, and it's also that at least with ... it's the guideline side of things for us. Like this is what you should be doing. But to your point, if you're doing it per industry, that it gets very complex. And also then to your point is that every industry might be slightly different. So therefore you have to find out which is the one that you need to probably standardize on. I remember one of those things like GDPR, I had advising banks in Africa and they had banks that most of their client base 90% were local citizens and 10% were EU. And they were going, do we separate the way we do our business? Do we do 10%? Do we do GDPR just for the EU citizens or we apply it to all? And they ultimately find that actually it was in their best interest to apply it to all because it made it much more easier.
It also made it much more transparent for them, much more controllable, much more visible. So in same way, I think that when you look at companies in the US that adhere to the California Consumer Privacy Act, took the same approaches. Well if I'm going to have to do it in California, I'm do it across the board, and it makes it much easier. So in the same way that AI is across industry, you're going to have that same approach. Definitely, I like the risk-based approach, for me is definitely allowing you. And also the more impact you have, the more you have to make sure that you're actually following the right practices and being more transparent and adhering to a lot of the regulations. So for me, I've always liked approaches that does consider the ethics and the human nature side of things because it might slow certain things down.
But I think the EU AI Act was a bit of a balance between one is allowing innovation, allowing things to move forward as long as you're not impacting the citizen life at the end of it, so you can actually move things and forward. So there has to be the balance between both, making sure that everyone's safe but at the same time, making sure that technology can thrive within the society. So what of these areas do you think that, which ones that should we be cautious about, which ones should we try to accelerate as much as possible? Is there certain areas that you think that we should slow down and be a bit more cautious onto or things that we should try to advance as much as possible when it comes to AI systems?
Andrea Isoni:
In term of technology where we should evolve?
Joseph Carson:
Yeah. In technology.
Andrea Isoni:
Yeah. Well I have the feeling that we are evolving quite fast any case, but, yeah.
Joseph Carson:
I mean when the CEO of Nvidia gets up and says we shouldn't learn to code, I'm just like, no, because it's the point who's going to fix the car when it breaks down?
Andrea Isoni:
Yeah, I think in media CEO say yeah, we not need coders or something like that. There are people that say that, I disagree on that.
Joseph Carson:
Who's going to create the algorithms?
Andrea Isoni:
Yeah, but the problem... Anyway, I disagree on that long copy of discussion to explain that, but yeah, no, we need people that be able to intervene and fix things-
Joseph Carson:
Absolutely.
Andrea Isoni:
... When there are issues or problem. Or integration because even if a code is well done et cetera, how do you integrate-
Joseph Carson:
Absolutely.
Andrea Isoni:
... Into even the integration automatically? Really?
Joseph Carson:
Yeah, interoperability. Interoperability is definitely one of the things that we're going to have to keep doing is how do we bring these systems together.
Andrea Isoni:
Definitely not soon. Definitely not soon because first of all there is a limit of line of text the alto can produce. And by the way, the more you a large number of tests, the number of error is nonlinear. I mean, risk of error, exponential increase is not the same number of levels. The large text if it's code or whatever, is increased nonlinear, no, you cannot possible do that way. I don't have a specific industry in which I think we are progressing too slowly in a way. I don't have that feeling.
Joseph Carson:
Yeah, definitely, I think that the chat bots are a bit like... The help desk and support where a lot of organizations are basically move to chat bots, which is sometimes a bit frustrating. You can start having arguments with them because at some point in time, I like the ones that have a bit of a balance that will help you so far and then move you to a person at the end.
Andrea Isoni:
I like the combination too is sometimes too difficult to explain to a chat bot what you want still. It's just quicker to go with a human because it's just quicker. Sometimes it's a bit difficult because obviously, they're standardized and things like that. By the way, there will be even more standardized after EU AI Act and the reason is they need to respect the regulation or avoid to be fine or things like that, which means that creative parts of this will be a little bit cut to be safe on the regulation perspective.
So when you say that we are not moving too fast in adoption, definitely is the case and five think the adoption of AI, it will pause or slow down at very least a bit until everyone is safe, as in safe from fine, safe from consequences from the regulation. I think the adoption is fortunately, unfortunately judge yourself, but it's slowing down. It has to slow down especially in sophisticated model because a company wants to be sure it's not going to be fine or it's not going to throw problem in that sense down the line.
Joseph Carson:
Absolutely. I think from my side is that what I've challenged with is the accuracy and high confidence level. Whereas a lot of the models, what they don't provide you is, is some type of transparency into, we have this much confidence that the answer we give you is correct. And that's what's missing a lot is a lot of these is that when you get a response back, you have to try and verify yourself whether the response that you got from basically the GPT model is accurate and how do you understand about what data sets, what training model was used or what. So for me, not having visibility into the accuracy model is something that I think that will provide the much more confidence and basically the acceleration side of things. Because what's happening is the more, if we start interacting with a model and all of a sudden, nine times out of 10 we get the wrong response, people will go somewhere else.
And I think that's ultimately is the correctness and accuracy is something that... I remember sitting, one of the panels I was on was about using AI in law enforcement and one of the things I made the statement was, is that when you do that, then you had to be right all the time. You can't have one error because the moment you have one error is that you invalidate everything else before and that's where you have the challenge. It's like somebody who's doing forensics and all of a sudden they've been working on hundreds of cases and you find out that person has done it wrong in one case, is that all of the cases had to be reviewed. So did they do the same mistake in all the previous ones?
So for me that's when you get into that situation is accuracy and correctness and confidence levels is we need to really emphasize the importance in those. Otherwise, once how it's been used, you might actually get in a situation where you could, all of a sudden, invalidate all the hard work we've done to that date plus also the confidence level of people in the output as well.
Andrea Isoni:
Absolutely. In fact, just to return on that, obviously there was no time discussed too much on that, but in the higher risk here, you need to provide evaluation metrics. They have tested the models, the accuracy of things like that. Somehow, somewhere you need to provide evidence that you have an evaluation make on that. And on top of that, ISO, International Standards Organization, they are new certification available exactly in the same way. Anyone's having a... in cybersecurity 27,001, quite famous one, there will be the same concept as in you need a management record, you need an incident record, you need procedures or processes or what, on accuracy.
Joseph Carson:
Correct.
Andrea Isoni:
On evaluation and accuracy and your model. And that's the way you're going to be certified in this case on artificial intelligence security.
Joseph Carson:
The outcome of that panel came to where when you're using it in law enforcement is that you have to have very, very clear explainability. What was basically the outcome of that type of subject matter expert discussion is that when you're using it for those certain cases, you have to have full explainability and the accuracy and the confidence level that you got to that outcome. So therefore, there is no question later is that you have full disclosure into that. So you're absolutely right, is having the transparency and having the models and having going through and showing all the possibilities is the explainability, which becomes important. What's your thoughts on the future? Where are we going? Let's say EU AI Act comes, do you think other countries are going to follow, other locations are going to try to model up to that? I think that we talk about protection levels of citizens around the world. It's getting very different. Some citizens are losing out, not just even from... It gets into challenges between future jobs and careers and economies. So where do you think this is going in the future?
Andrea Isoni:
Yeah, first of all, in a business perspective, as I just mentioned briefly before, I think AI are ... anyway, the models that you see in real life and the sophisticasy of that and the creativity of that will be a little bit pushed down. As I said before, they are scared of being... I give an example, they to understand ChatGPT version four. When was first released was amazing creativity as in was very, very, very creative. You can find already, by the way. And then at some point they put down the generation... Was still very good, but the generation creativity way was a little bit tuned down. The reason is... That's my opinion, I have no proof of that. But the reason is yes, it was great, but also was great in the bad way. It was go wrong in two sides. It was great for people that using well, but also when it was wrong it was impacting.
Joseph Carson:
It was very wrong.
Andrea Isoni:
So they tune down, the generation, the creative ways somehow, somehow somewhere, its not a topic for today, but the reason is in my opinion, they need to be within safe standard from the regulation perspective. So if anything, this regulation will push down, not up, push down. Fortunately, the sophisticacy of the model, the creativity of that because it needs to obtain certain safety order obviously to avoid fines or things like that of a business perspective. That's the first thing. So we are not going to be up in term of advancement too much, but I think ADA stable a little bit down for that reason. Second on, well EU AI Act will be followed by other things like that. It depends on that. I think many country will tend to be light touch as in, but the light touch reason behind this A; the example of flight tickets working on that, do really need an essential regulation?
If it's already protected, we don't. But as I was trying to explain is it's not clear to me yet is okay, we don't need this specific overall encompassing regulation just for AI. We just need to push a sector. Now, the problem is if you do buy sector, it is really less laborious for a company or not. That's exactly the tricky question because somehow somewhere we found out that it's counterintuitive. They started for the good reason, as in, we do not want business to in their developmental adoption, et cetera. But if it turns out the opposite, as in everyone should be not regulating any single industry, it backfires.
Joseph Carson:
And the inconsistencies as well.
Andrea Isoni:
It backfires because they started for that. But then you're right, the EU AI will be the way.
Joseph Carson:
Yeah, absolutely.
Andrea Isoni:
But if it's not, I don't think we'll be too far followed, but at this very moment in time, I'm not sure which way is-
Joseph Carson:
I think some subjects are sitting on the fence.
Andrea Isoni:
Essentially...
Joseph Carson:
They're just sitting on the fence right now and waiting to see.
Andrea Isoni:
I don't know which one is... At the end of the day, I think every country it wants the best compromise. You want security for the system, but at the same time, don't diminishing this-
Joseph Carson:
Innovation.
Andrea Isoni:
... Company. Yeah, the innovation level of your own company.
Joseph Carson:
Absolutely.
Andrea Isoni:
That's the main topic. Maybe not in the EU, but most country thinking that way, if not all. So how that can be achieved, we need to see, it's not straightforward as I say, the UK approaches that one there is less cumbersome on the company, but if there is a way, as you say, standardized this, maybe will be that. If not, the EU AI kind of approach will be the way.
Joseph Carson:
Absolutely. Andrea, many thanks for being on the show. It's been fantastic discussion with you and hopefully this will be the first of many conversations. This is something that, a topic I really enjoy and I think it's very exciting, the possibilities in the future. But any final words that you'd like to leave the audience? The audience would like to reach out and contact you for more information, what's the best way to contact?
Andrea Isoni:
Sure. I'm very active on LinkedIn. I reply to everyone, really. So when they find me, Andrea Isoni on LinkedIn. And yeah, from there I really replied to everyone... and I-
Joseph Carson:
Fantastic.
Andrea Isoni:
... I discuss all this topic there with a newsletter on AI called...
Joseph Carson:
Fantastic. I'll make sure that we have the way to contact you on our show notes so that it makes it much easier for everyone. Andrea, many thanks for joining with the show today and hopefully for the audience, this has been something very valuable, really getting into some of the hot topics of AI and some of the directions and some of the good paths is going, some of the more cautionary side of things, the ethics, and as well as looking into the EU AI Act.
So, many thanks everyone. Take care, stay safe. Andrea, again, many thanks for being on the show. And for the audience every two weeks, tune into the 401 Access Denied Podcast and for thought leadership news, hot topics and exciting information that really helps you understand some of the best way forward. So thank you. Stay safe and take care.
Andrea Isoni:
Bye.