The rising danger of AI poisoning: When data turns toxic
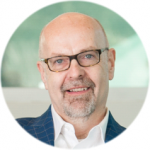
Tony Goulding
Imagine deploying an AI system designed to enhance cybersecurity, only to discover that it’s been covertly manipulated to overlook critical threats. This scenario isn't a distant possibility, but a present danger posed by AI data poisoning attacks.
If you have responsibility for protecting the AIs in your organization, you’ll want to read on to learn how to do your job more efficiently and effectively. It’s critical that red teams and advanced security professionals understand AI poisoning techniques, how they work, and the risks they pose.
This blog will help you pressure-test your security controls and practices. SOC teams, incident responders, and security engineers may not need deep technical knowledge of data AI poisoning and other AI-related threats, but it’s still important that you understand how to identify and mitigate vulnerabilities that could lead to AI poisoning.
In addition, IT administrators, Identity and Access Management/Privileged Access Management teams, compliance pros, and security leadership must also increase their awareness of this mounting issue so they can partner with those on the front lines. AI poisoning can impact the work of other IT, risk, product development, and business teams, which is why it’s important to increase awareness of this subject.
I've attempted to answer questions about AI poisoning that are likely on your mind through the lens of identity security.
What is AI poisoning?
AI poisoning is a tactic that undermines the integrity of AI models by manipulating their training data or processes, leading to compromised performance or unintended behaviors.
AI poisoning attacks often play out by leveraging enterprise identities and credentials. Illicit access to the systems that host AI applications and their data sets create blind spots akin to third-party access from an extensive software supply chain.
AI models rely heavily on extensive datasets for training. For instance, OpenAI's ChatGPT-4 comprises eight models with almost two trillion total parameters. Managing such vast pipelines introduces risks to interconnected systems, services, devices, and the AI models themselves. Attacks can occur during data collection, storage, and preparation.
A poisoned medical AI could misdiagnose patients, while a compromised financial model might make erroneous investment decisions
AI poisoning attacks can have severe consequences, especially when AI systems are deployed in critical sectors like healthcare, finance, or autonomous transportation. For instance, a poisoned medical AI could misdiagnose patients, while a compromised financial model might make erroneous investment decisions.
The AI poisoning playbook
Adversaries use three main AI poisoning techniques.
1. Data Poisoning:
Attackers insert malicious data into the training dataset, causing the AI model to learn incorrect patterns. This can result in the model making consistent errors when processing specific inputs. For example, altering traffic sign images in a dataset could lead to misclassification by an autonomous vehicle's recognition system.
2. Model Poisoning:
In collaborative training environments, adversaries can inject harmful updates into the model during the training phase. This manipulation can degrade the model's overall performance or implant specific vulnerabilities. For instance, consider a collaborative fraud detection system used by multiple banks. Each bank trains the AI model locally and then submits updates to a central server, aggregating them into a global model. An attacker who gains access to one of the contributing nodes (e.g., a compromised bank's server or a rogue insider) can inject subtly altered gradients that introduce vulnerabilities into the global model.
3. Backdoor Attacks:
Attackers embed hidden triggers within the model that, when activated by specific inputs, cause the model to behave unexpectedly. For instance, an AI-powered facial recognition system is used for access control at a secure facility. The AI model has been trained on a dataset of employee faces to grant or deny entry based on identity verification. An attacker poisons the training data by inserting specially crafted images that introduce a hidden backdoor trigger—a small sticker, eyeglasses, or unique pattern placed on a person's face. The model learns to associate this specific trigger with "access granted," regardless of who wears it.
AI models that rely on large, diverse, and externally sourced datasets are the most susceptible. Models that lack strict data integrity controls, operate in open environments, or are used in high-stakes decision-making face the highest risk.
AI and identity security work together
AI, like traditional business applications, requires permissions to function. Those permissions come from machine identities assigned to AI applications and services (AI identities.) However, without robust identity security measures, these AI identities can become vectors for attacks:
Credential Compromise: If an AI system's credentials are not securely stored, attackers can hijack them to manipulate the system's behavior or access sensitive data.
Unauthorized Access: Without strict access controls, malicious actors might exploit AI identities to introduce poisoned data or alter training processes.
As use of AI agents and LLMs increase in your organization, your identity security strategy must adapt to protect them.
Luckily, you can leverage the capabilities of AI that are built into identity security solutions to help you do so.
How can identity security block AI poisoning attacks?
Preventing AI data poisoning requires securing the sources of training data to block unauthorized modifications before they occur. A Zero Trust identity security approach, combined with strict identity security controls, ensures that only verified and authorized entities can access and modify data used for AI model training.
The focus here is on strict identity management, since compromising privileged identities will be the primary tactic used by adversaries to gain access to the systems this data resides on. Another focus is layering controls on workstations and servers to protect those systems by enforcing least privilege.
Identity Governance and Administration (IGA) and Governance, Risk, and Compliance (GRC) establish baseline permissions by managing role-based access policies, ensuring that only authorized users and services have the minimum necessary access to AI training data and models while maintaining auditability and compliance.
A Privileged Access Management (PAM) vault will protect access to privileged user and machine identities that would give an adversary access to systems in your AI infrastructure. PAM access controls on workstations and servers will enforce the IGA policies to ensure only valid identities have access. This can be augmented with multi-factor authentication (MFA) and just-in-time (JIT )access request workflows for additional user validation.
How can identity security detect if AI models or datasets have been poisoned?
Detecting AI data poisoning often relies on statistical analysis, anomaly detection, and adversarial testing—methods outside the scope of identity security. However, these techniques do not address how attackers gain access to manipulate data in the first place.
Identity security helps by restricting unauthorized access to training data, models, and pipelines. PAM enforces least privilege, ensuring only authorized users can modify AI assets. Identity Threat Detection and Response (ITDR) flags unusual access patterns, such as privilege escalation or unauthorized dataset modifications, that may indicate poisoning attempts.
Segregation of Duties (SoD) reduces insider risks by ensuring no single user controls both data ingestion and model training. Governance Risk and Compliance (GRC) maintains audit trails, providing forensic evidence in case of a poisoning incident.
What role does data governance play in preventing AI poisoning?
Data governance plays a critical role in preventing AI poisoning by establishing policies and controls that ensure the integrity, security, and traceability of data throughout its lifecycle. It enforces strict access management, so that only authorized users can access systems where AI applications and training dataset files reside. By integrating IGA and PAM, you can enforce least-privilege access, preventing unauthorized data modifications that could introduce poisoning.
Also, data governance mandates audit logging and version control, ensuring that all dataset changes are recorded and traceable and to detect and roll back unauthorized or suspicious modifications before they impact AI model training. SoD further reduces risk by preventing any single individual from controlling both data ingestion and model development.
By aligning with GRC frameworks, data governance also ensures that AI data sources adhere to industry standards and regulatory requirements, minimizing exposure to poisoning attacks.
What should you do if you suspect your AI models have been poisoned?
If you suspect AI model poisoning, take immediate steps to contain the threat, analyze its impact, and restore model integrity. The first priority is isolating the affected model to prevent further damage, including halting its deployment if it is actively making decisions. Don't risk poisoned outputs propagating to critical systems.
Next, you should conduct a forensic analysis of training data and model behavior. Using ITDR can help your security teams investigate unauthorized access patterns, privilege escalations, or unusual modifications to datasets that may indicate how poisoning occurred. PAM logs, session recordings, and audit trails can help identify whether an insider, a compromised account, or an external adversary was involved.
If poisoning is confirmed, rolling back to a known good dataset and model version is essential. You can leverage GRC capabilities to help verify data integrity and ensure compliance with security policies before retraining the model. SoD should also be reviewed to close any gaps that may have allowed unauthorized changes.
Finally, reassess your preventative controls and if suspect, implement strong ones, such as enhancing access restrictions, enforcing more rigorous data validation processes, and integrating automated monitoring to detect future poisoning attempts before they impact AI models.
What countermeasures mitigate the effects of AI poisoning?
Mitigating the effects of AI poisoning requires a combination of proactive security controls and reactive response strategies.
For proactive measures, IGA ensures that identities have the appropriate access through lifecycle management, role-based access control (RBAC), and access certification, preventing overprivileged accounts before they become a risk. GRC enforces security policies, compliance frameworks, and audit controls to ensure identity-related risks are managed in alignment with regulatory and organizational standards.
SoD prevents conflicts of interest by restricting users from having excessive control over critical functions (e.g., preventing a single user from modifying AI datasets and training models). Cloud Infrastructure Entitlement Management (CIEM) continually assesses and enforces least privilege across cloud environments by identifying and remediating excessive entitlements to prevent privilege misuse. PAM controls and restricts high-risk access to critical systems, enforcing just-in-time access and session monitoring to reduce attack surfaces.
For reactive measures, ITDR monitors access behaviors, detects anomalies, and responds to identity-based threats such as credential compromise, privilege escalation, and unauthorized access attempts.
How can adversarial training improve your AI poisoning defenses?
Adversarial training is valuable by exposing your AI models to manipulated inputs during training, helping the model learn patterns that distinguish normal data from maliciously modified inputs. However, this doesn't prevent attackers from poisoning the dataset in the first place, it only helps mitigate the impact. This is where identity security comes into play with preventive and reactive measures as described above.
How can you balance AI adoption with security risks?
You must understand both sides of the AI coin as described earlier and integrate identity security controls into your AI workflows.
AI development requires access to vast datasets, cloud environments, and compute resources—all of which expand your identity attack surface. Identity security measures must be adaptive and automated, allowing AI teams to work efficiently while preventing threats such as data poisoning, unauthorized access, and privilege abuse.
This is not a new journey, however, as it has parallels with the use of identity security in DevOps environments.
How will AI poisoning attacks evolve in the coming years?
That's a crystal ball question. But, based on how rapidly AI has evolved over the last few years, we can anticipate attacks to become more sophisticated, targeted, and automated as adversaries refine their techniques and exploit new AI vulnerabilities. As AI becomes more embedded in critical decision-making, threat actors will increasingly discover and exploit vulnerabilities.
Some areas to watch from an identity security perspective include:
- Using AI to make their attacks stealthier and better at avoiding detection. ITDR will need to account for this.
- More automation in attack scenarios. Using ML trained to generate optimized poisoned data to maximize disruption while minimizing detection. SoD, PAM, and ITDR play a role in this.
- Targeting upstream organizations in your supply chain as you rely more on 3rd-party datasets and pre-trained models. GRC to help with provenance tracing and integrity checks could help.
- Poisoning-as-a-Service. Following the lead from Ransomware-as-a-Service, we can expect service models that provide such tools for rent. CIEM could help by restricting access to cloud-based AI training pipelines, preventing threat actors from injecting poisoned data.
- Hybrid attacks. Today we see attackers focus on compromising privileged accounts to gain access to sensitive data. Attackers will likely extend this playbook to include AI data as a prime target. PAM, ITDR, and least privilege models like Zero Trust will be essential here.
Risks and impact on my organization
What are the risks of AI data poisoning for enterprise environments?
It depends on the role of the AI. Increasingly, AI models drive automation, fraud detection, cybersecurity, and business intelligence. So, poisoning attacks can have long-term and widespread consequences, more so if left undetected.
Which business sectors are most at risk of AI poisoning attacks?
Any industry that heavily relies on AI-driven decision-making will be at a higher risk. Those where data integrity is critical with direct consequences on security, financial stability, or public safety might be considered the most vulnerable.
How can poisoned data affect AI-driven cybersecurity tools?
Like any other AI model, AI used in cybersecurity tools could be a target for data poisoning. This could result in weakened threat detection, compromised biometric and authentication controls, increased false positives and negatives, and general exploitation of AI-based defenses.
Successful attacks could systematically degrade security operations, allowing attackers to evade defenses and disrupt operations. It's incumbent on identity security vendors to address both sides of the AI coin by ensuring AI-augmented capabilities built into their products are protected against such attacks.
What are the financial and reputational consequences of AI poisoning?
AI poisoning attacks can result in severe financial losses and reputational damage, particularly for organizations that rely on AI for fraud detection, cybersecurity, healthcare, finance, or critical infrastructure. As discussed above, poisoned AI models can generate faulty decisions, introduce security vulnerabilities, compliance violations, and customer distrust, leading to direct financial costs and long-term brand damage.
Could AI poisoning be used to manipulate fraud detection, insider threat monitoring, or privileged access management (PAM) solutions?
Yes, AI poisoning can be weaponized do this, potentially weakening security controls, enabling unauthorized access, or generating false alerts. For security solutions that rely on AI-driven behavioral analysis, anomaly detection, and access pattern monitoring, poisoned training data can redefine what is considered normal or malicious behavior, allowing attackers to bypass security or disrupt operations.
What emerging compliance and industry standards are addressing AI poisoning?
Currently, there are no industry standards or regulations that specifically address AI poisoning attacks. However, several emerging initiatives and frameworks aim to enhance the overall security and integrity of AI systems, indirectly mitigating the risks associated with data poisoning:
- In the U.S., NIST has established the AI Standards Coordination Working Group to promote effective federal policies leveraging AI standards.
- Internationally, the ISO has developed standards pertinent to AI governance, such as ISO/IEC 42001, ISO/IEC 23053, and ISO/IEC 22989
- Various regions such as the U.S. (California specifically with SB 1047) and the E.U. are formulating AI regulations that, while not explicitly targeting data poisoning, encompass broader AI safety and security concerns
- Organizations and industry consortia such as Anthropic, the AI Safety Institute Consortium, the Cloud Security Alliance, the NSA, and CISA are proactively developing best practices and guidelines to bolster AI security
Delinea reduces your risk of AI poisoning
Delinea provides a comprehensive approach to safeguarding AI systems, ensuring their integrity and reliability in an increasingly digital world.
Delinea’s identity security platform includes access control and privilege management, along with AI-driven threat response and adaptive security enforcement, to:
- Better detect anomalous activity across the entire infrastructure.
- Analyze identities, roles, rights, and access patterns across your identity fabric (including identity providers) to assess and prioritize identities based on their risk.
- Consolidate intel from PAM, ITDR, CIEM, IGA, and GRC to provide a more accurate and complete assessment of identity risk posture.
- More accurately detect anomalies through better model training. This will result in fewer false positives and more trust in automated remediations such as account disabling or dynamic policy updates.
- Enhance JIT access decisions with AI-based risk scoring to automatically adjust privileges and duration based on behavioral risk levels instead of static policies.
Learn more about the Delinea platform and how it can help you combat AI poisoning.
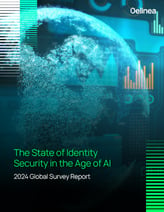